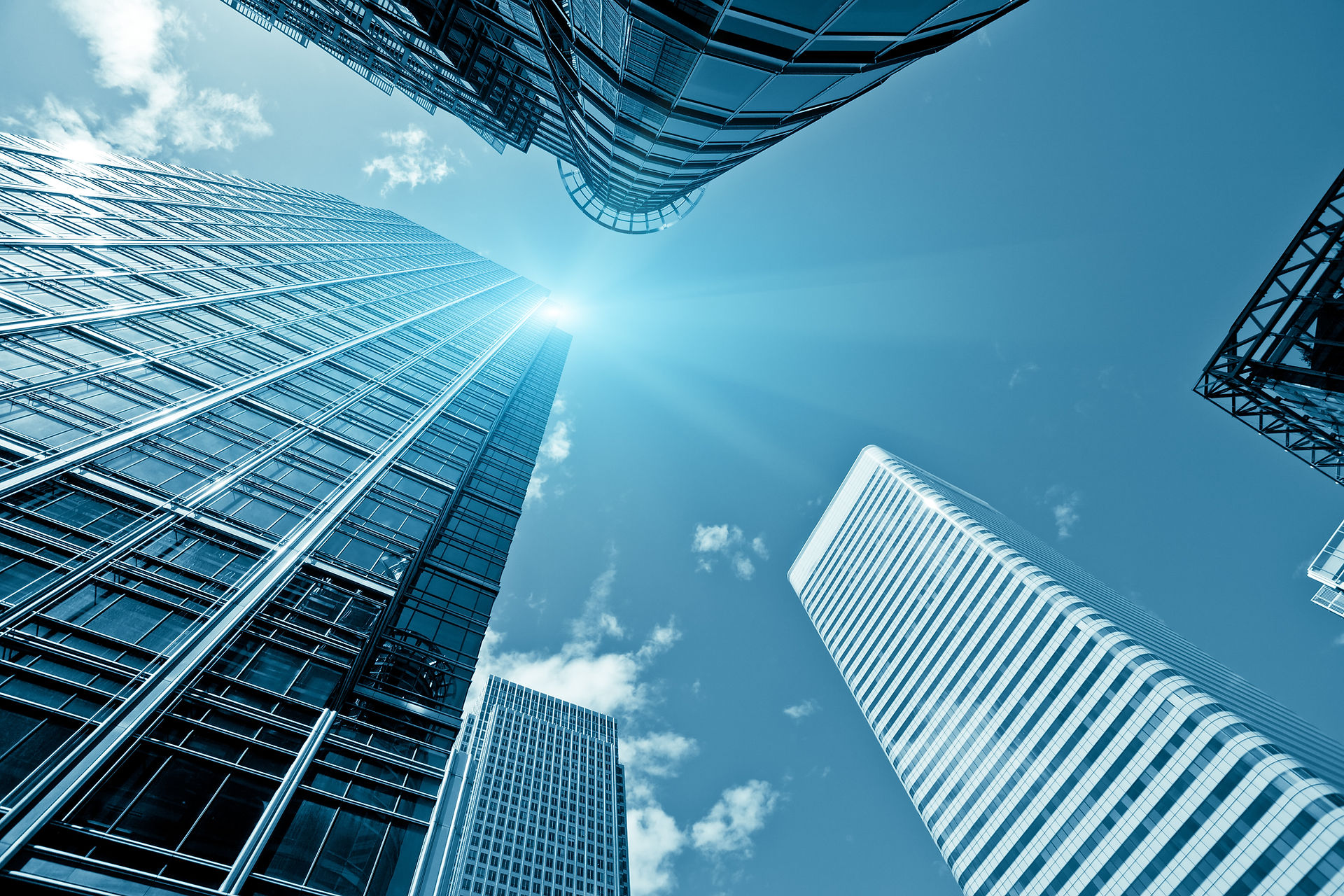
Building Energy Assessments, Solutions, and Technologies BEAST
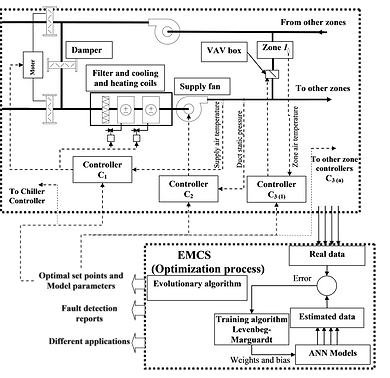
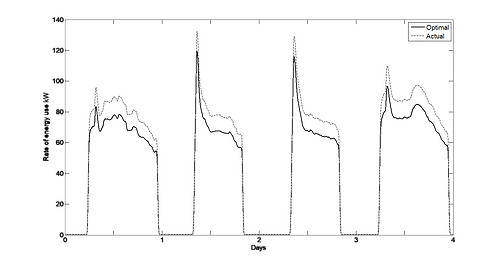
Modeling and Optimization of HVAC Systems Using Artificial Intelligence Approaches
Intelligent energy management control system (EMCS) in buildings offers an excellent means of reducing energy consumptions in HVAC systems while maintaining or improving indoor environmental conditions. This can be achieved through the use of computational intelligence and optimization. The work thus proposes and evaluates a model-based optimization process for HVAC systems using evolutionary algorithm and artificial neural networks. The process can be integrated into the EMCS to perform several intelligent functions and achieve optimal whole-system performance. The proposed models and the optimization process are tested using data collected from an existing HVAC system. The testing results show that the models can capture very well the system performance, and the optimization process can reduce energy consumption by about 11% when compared to the traditional operating strategies applied.
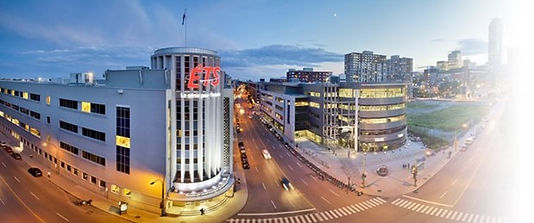
Chilled-Water Central Plant Modeling
Chilled water systems account a large portion of buildings total energy use. It is essential to find an optimal approach to reduce the energy consumption of a building by incorporating system model and optimization methods to create a model that can accurately stimulate the performance of the system, using real time data from the chilled water system. The proposed technique
develops a data driven system model by using machine learning techniques to predict the energy consumption of the system. It also introduces an optimization method for model structure parameters. Incorporating a data driven model and the optimized model structure parameters result in a more accurate model that can be used in a wide variety of applications such as fault detection and diagnosis, measurement and verification assessment to ensure a fault free system. The objective function is to reduce the error between the predicted value by the model and the actual system output. The optimization variable is model parameters and structures such as optimal number of neural network cells, optimal time delay and etc. Several machine learning modeling methods such as artificial neural network, KNN and bagged tree were used to develop, train and test models. The result of incorporating a data driven model and the model structure optimization tool shows that the optimized model can capture and predict the performance of the chiller with a higher level of accuracy (10%-20%), compared to the non-optimized model with R2 value of more than 0.99 which can potentially be used alongside with other optimization techniques to ultimately reduce the energy consumption of the buildings.
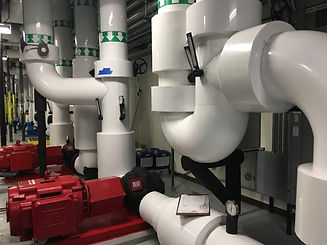
Chilled-Water VAV Systems Modeling
The most important factor in achieving optimal performance is the need for accurate models to predict the whole-system and component performance. This research proposes data-driven modeling and optimization techniques to accurately predict the performance chilled-water air handling units. The work investigates several data-enabled modeling techniques such as artificial neural networks and ensemble of bagged decision trees and propose model improvements through model structure optimization. The optimization algorithm will determine optimal model structures such as number of neural networks, time delay, Feedback delay, and Bootstrap Aggregation algorithm. Performance data were collected form real buildings to train and test the models. The optimization problem is solved using Genetic algorithm to reduce the error between the simulated and actual data for testing period. The models predict the supply air temperature as a function of (1) chilled water temperature, (2) chilled water valve position, (3) mixed air temperature, and (4) supply air flow. The testing results shows that the proposed models can predict accurately the system performance. The R^2 of the testing results that measure the model accuracy level was within 95-99% depending on the model structure and type. Moreover, the use of such data-driven models can help in developing several aspects of the industry such as better real-time control, fault detection, optimization of the system performance, and help reduce the overall energy consumption
.
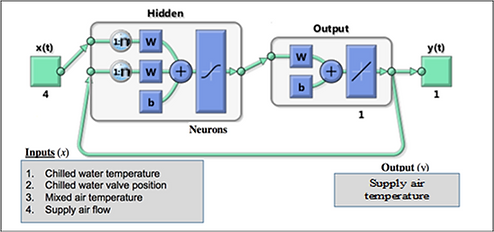
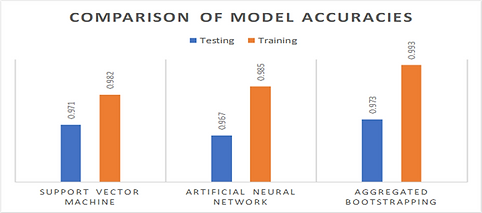
Optimization of Chilled Water Plant Design
Chilled water central plant accounts a large portion of total energy use and cost in building. The chilled water central plant design will have a significant impact on this energy cost. This work proposes a multiple objective design optimization method for optimal design of chilled water central plants. The method integrates whole system models with multi-objective genetic algorithm optimization solver to minimize the annual energy cost, initial cost, the life cycle cost, or any combination of those costs. The design variables considered are chilled water and condenser water piping diameters, chilled water supply temperature, and condenser and chilled water temperature differences. The proposed approach combines cooling load analysis and head and energy calculations integrated with whole chilled water plant model. The pump head calculations including piping, all fittings, valves, and devices are achieved by developed chilled and condenser water flow model. The energy calculations are done by using generic chiller, fan, and pump models. The method is tested on an existing three-story, eighty-eight thousand square foot building. The annual energy cost vs. initial cost, and initial cost vs. life cycle cost were selected as two objective functions to be solved by two-objective GA optimization algorithm to obtain a set of solutions for better design decisions. A whole building energy simulation model is used to generate the hourly cooling loads and then the optimal design variables are found to minimize the two objective functions. The testing results show this approach will achieve better results than rules-of-thumb or traditional design procedures. The life cycle cost saving could be up to 8% depending on project specifications and locations.


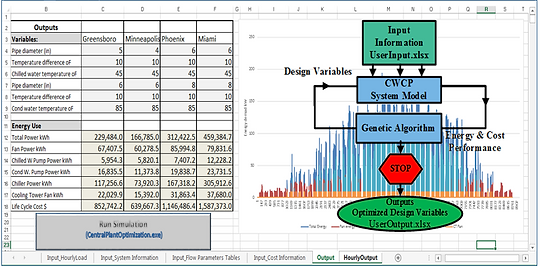
Developing and Testing of Single-Speed DX Air Conditioning System Model
This work intriodcues the development and testing of a single speed DX air coil model to predict compressor power, heat capacity, coefficient of performance COP, and supply air temperature and humidity ratio as a function of airflow rate, air conditions entering the coil, and outside air temperature. The proposed model is calibrated using nonlinear regression analysis of online data collected from a typical building automation system if installed, short time data measurements, or manufacture’s data. The model parameters are determined through nonlinear regression instead of traditional methods with parameters defined at rating conditions.
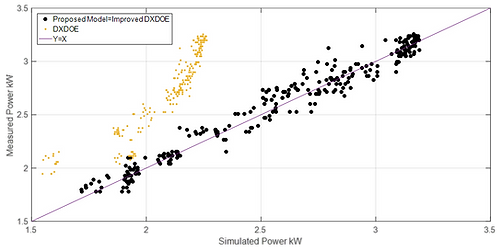
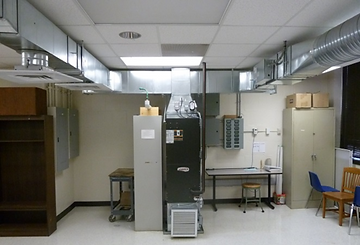

​
To test the model, experiments were conducted on a 3-ton DX split system air conditioning unit fully instrumented. The unit ran over the course of several months to get a wide range of operating data. The testing results showed that the model can accurately capture the performance of DX air conditioning system as compared to the existing EnergyPlus DX model. This proposed model can be a powerful tool to be used for serval intelligent applications such as energy assessment, fault detection and diagnosis, and system performance optimization.
Developing Regression Models for Estimating Monthly Energy Consumptions in Schools in Hot and Humid Climates
Modeling of energy use in existing buildings is required for different applications such as building energy management, establishing baselines, and calculating retrofit savings. A single or multivariate regression model is mostly used as a means of developing these models of energy consumption in buildings. This work explores the applications of single and multivariate models on estimation of monthly energy consumptions in schools and discusses the several aspects of model improvements. Several regression models ranging from a simple only outdoor dry-bulb temperature to multivariate models are studied to find a comprehensive methodology in estimating energy consumptions in schools located in hot and humid climates. Data on energy consumptions in 225 schools selected from four different counties in central Florida are collected to evaluate the models and provide recommendations for improvements. The results show that by including the school scheduling in the model, the accuracy in the monthly energy consumption prediction is significantly improved comparing to that when using only temperature regression model.
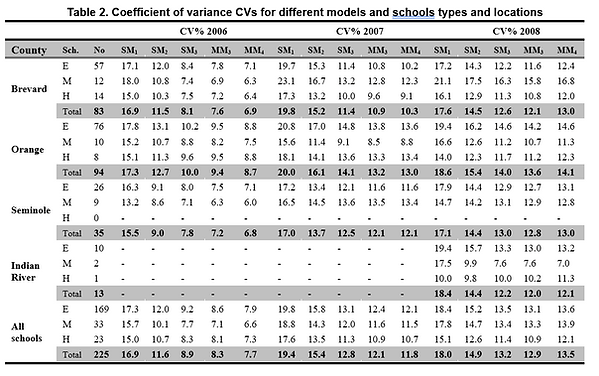
Study the Impacts of Air Filters on Energy Consumption in Typical HVAC Systems
Filters are used in HVAC systems for both commercial and residential buildings to protect the equipment and improve indoor air quality in conditioned spaces. Although there are many benefits of using the air filter in an air conditioning system, the resistance associated with it can increase fan energy use and may adversely affect air conditioning system performance and efficiency. The paper explores the impact of air filtration on energy consumption for a typical air conditioning (AC) system with constant or variable-speed fan. A whole building simulation model is used to simulate the annual energy consumption for various air conditioning system capacities, different levels of filter cleanliness, and various filter minimum efficiency reporting values (MERV). The results indicate that with a constant-speed fan, the cooling energy use increases as the filter gets dirty over time and the energy use in the fan may increase but this depends heavily on the investigated fan performance curve. With a variable-speed fan, the fan energy uses increases with a dirty filter but the cooling and heating energy uses are slightly affected. The fan energy use rise due to the dirty filter depends mainly on air system capacities, filter MERV ratings, and the degree of the filter cleanliness.
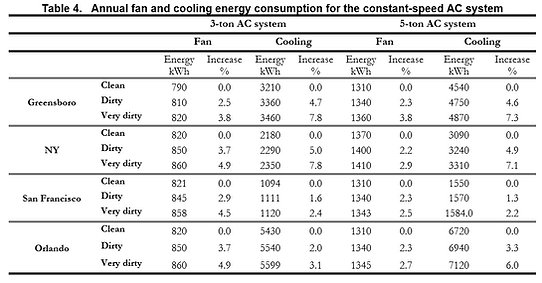

Develop of Supply Air CO2-Based Demand-Controlled Ventilation for Multi-Zone HVAC Systems
The work discusses a new ventilation control strategy based on supply air CO2 concentration for multi-zone HVAC systems. The strategy offers a great opportunity to reduce energy consumption in HVAC systems while providing the required ventilation according to ASHRAE standard 62.1. The strategy requires a PI/or PID controller along with a CO2 sensor located in the main supply air duct to control the supply air concentration by adjusting the outdoor air damper. It also includes a control algorithm to dynamically reset the CO2 concentration set point on a proper time interval basis and using the actual zone airflow rates. The strategy is evaluated by simulations on multi-zone office building conditioned by a typical VAV system and under various USA locations. The results show that a significant saving could be obtained by implementing the proposed strategy as compared to the design-occupancy ASHRAE Standard 62.1 procedure and this saving depends mainly on actual occupancy profile and building locations
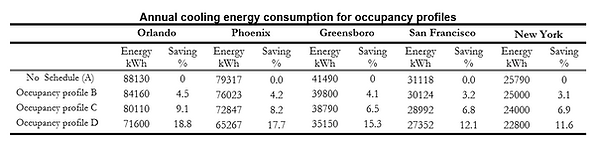
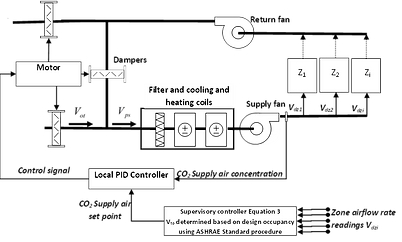